The ML Playbook Series: A Conversation with Tom Whitehead
3rd September, 2024 10 min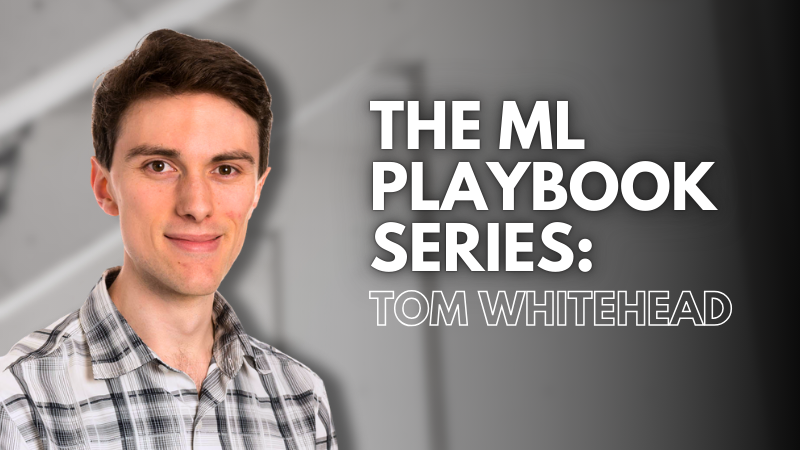
Welcome to the third edition of The ML Playbook blog series, where we delve into the insights and experiences of industry experts shaping the landscape of Machine Learning.
Our third guest, Tom Whitehead, is a seasoned Head of Machine Learning, having been at Intellegens for nearly seven years focusing on applied machine learning for the materials, chemicals and manufacturing space.
Tom obtained an MSc in Physics from the University of Oxford and a PhD in Theoretical Physics from the University of Cambridge. I had the privilege of having a chat with him around all things ML as well as specifically diving into the R&D side too.
What inspired you to transition from theoretical physics to practical applications of machine learning in industry?
So I guess for me it was, how do I make an impact? I wanted to see some of the impact a bit more directly than you typically do in academia. And machine learning was good for that because it's a good place to transition from the sort of quantitative physics that I was doing. Machine learning is fundamentally just maths and statistics, it's the same sort of idea.
For the industry side, that impact part, how do you make something that someone, some human will actually use? If you help someone design a product, and then someone goes and buys that thing and gets value from it, the impact is very direct, both for me and our customers.
Building on that, for those looking to bridge the gap between academia and industry, what advice would you offer to successfully transition and make an impact in practical applications of machine learning?
My advice, and the way that I've certainly gone about it, is to change one thing at a time. If you know something really well and you want to move in a different direction, don't necessarily drop all of that and jump straight to something new, but add on other angles. In my journey it was physics and then there was a little bit of consultancy, which was applying physics-based ideas to a different industry, and then the machine learning started as the tool that we're using to do that. It grows from there and you can slowly transition across.
I'm sure there are people who could jump from one thing straight to something completely brand new. But that's not me. I’d suggest learning new things and adding them to what you already know, so that you've got that good foundation there already.
Out of interest, do you think having a PhD or, or at least an advanced degree like a Masters, helps in what you're doing, especially in machine learning?
My experience in academia is only to PhD level, and at that level, what it's teaching you is how to learn quickly. It’s teaching you to skim a paper, pick up the key parts, and then file it away as “this will be useful one day / this won't be so useful one day”. And those are really helpful skills for the type of work that we do at Intellegens, which is applying ML to lots of different industrial problems, because you need to very quickly be able to skim the problem and understand what's the important part, what's the area of this problem that I'm going to attack. You don't need to understand all of it. In fact, you can't understand all of it. Someone else spent 50 years learning how to understand this problem, we're trying to come in and help them solve it very quickly, but we only need to understand the bit that we should focus on and how we can do that. I think that's something academia really brings or teaches you.
So I think having that academic experience does help for the way that we work at Intellegens, we need to be able to, fairly quickly, understand our customers’ pain points, which are the bits that we'll need to work on. I’ve found that the people who've brought the most to the team are doing that and yeah, they have always had either advanced degrees or a lot of practice. Someone who's brought in that experience from somewhere else is also great, but the PhD can definitely give you a good boost on that.
What skills do you believe are most critical for success in machine learning today, and how can professionals keep themselves updated with the rapidly evolving field?
In my opinion if you want to get into machine learning or you want to apply skills there, it's the hard skills at the bottom that are important. There's a bit of statistics, a bit of understanding; what does a machine learning model do, for example how does a neural network fundamentally work? And then you can apply that really widely. You can do whatever you want with that solid foundation.
In terms of keeping up with the ever-evolving field, I'm not sure I'm necessarily the best at that, but I get an email every morning that goes through arXiv and looks through papers that are in fields that I've tagged as being relevant. Some days, I'll go and skim through those and read the abstracts. See what people are talking about. I'll read one of the papers quickly and try and get that top level understanding and then if a customer comes on that it'll be relevant for, or something else that I'm interested in, I’ll go deeper, but yeah, just trying to get that top level understanding initially.
Having been the Head of Machine Learning at Intellegens for over six years, how have you seen the field of machine learning evolve, and what are some of the most significant changes and advancements you've witnessed during this time?
The big change is definitely ChatGPT, which didn't change anything fundamentally in the way we work at Intellegens, but what it did change is that the rest of the world was paying a bit more attention. We'd been working in AI and ML for years and people who understood the value that we're bringing saw that, but a lot of people were coming in fairly fresh to it. ChatGPT changed all of that and AI jumped into the mainstream. So that has been interesting because people think that they know what AI is, but the problems that we're solving at Intellegens are quite different to that. But at the same time, you've got that opening level of understanding there, which is quite nice. It's definitely a big change, let’s put it that way, but if it's net positive or negative, we'll find out.
Collaboration with R&D teams is a significant part of your role. How do you foster effective collaboration and ensure that the machine learning solutions you develop are aligned with the needs of these teams?
I guess this is again something that maybe Intellegens do a little differently than others which is that our team of people who go out and talk to the customers about their technical problems are scientists who've learned ML rather than ML professionals who are trying to learn science. And we've definitely found that it helps build a better rapport with our customers. If we can speak science to them, which is their language, and then translate that into the ML, rather than the customer having to do that translation, that works much better.
What trends do you currently see as the most transformative in the field of machine learning, particularly in the context of materials and chemicals R&D?
I think this goes back to what we were discussing earlier – ChatGPT – because of it, companies are understanding that data is something valuable they have and they should be using it, extracting that value out. A few years ago, it was more us at Intellegens saying “You should definitely utilise your data, it is valuable.” Now more people are coming to us and saying “We have data we'd like to get the value out of. Can you help us?” So that's really good for us, it's really positive, and it’s quite a big transition in the sense people are understanding it a bit more. Not everyone is 100% bought in and but I'm definitely seeing that it's coming more from the top level as well. Companies saying, “Hey, we're a big multinational chemicals company. We've been going for 100s of years. We have huge amounts of data. Why are we not using it?”
How do you see the role of machine learning evolving in the manufacturing sector over the next decade?
It's always dangerous making predictions, isn't it? In manufacturing in particular though, what I suspect it will be is embedded IoT devices. I think the manufacturing sector is moving more towards small models, very specialized that are designed for that one particular bit of kit that they're stuck on the side of. They’ll be very easy at point of use, which means it's a button or a little screen which is green (everything's good) or big red cross (don't do this - something's about to go wrong). A fair amount of the UK's carbon emissions comes directly from manufacturing sector, so if we can cut that, even by 1%, by using the AI embedded IoT devices that’s a massive win, an enormous impact.
Balancing strategic thinking with hands-on technical work is crucial in your role. How do you manage this balance, and what strategies do you use to ensure both aspects are effectively addressed?
I guess I’m pretty old school in that I've just got a to do list, I use Google Tasks to get things prioritised. I shuffle my tasks up and down as priorities change.
The management aspects of the of the role is trying to create an open atmosphere where people can always talk to me and each other, so I'm always on Slack or in the office, people can always come up and ask me if they need anything. Being available is something that I think is actually quite important for building your team cohesion, which is something we've got a really good version of here. The people are great, and they all like working together and collaborating. In terms of the head down hands on side of things, I typically work at home on a Friday: we have the morning stand up and then don't tend to have that many meetings during the day, so it's good for me to get some concentrated stuff done.
With your experience in startups and software solution development, what are some of the key lessons you’ve learned about driving innovation and managing teams in a fast-paced environment?
Always understand the objective before you start, understand the problem you're trying to solve before you actually dive in and start doing stuff. It's very easy to solve a really interesting problem that is not what the customer’s interested in, which is therefore not much use.
There's also a tension between doing a perfect job and doing something quickly that's going to generate the actual value. We're a small team and we're not venture funded, so we have to generate value for our customers so that they stay our customers and that's getting results out quickly, getting it out in their hands, so they're generating value.
This is where having the right people on the team, people who get on with each other and get on with the customers. If you've got great people who work together well, which I think we do, then you can get anything done, really.
So would you say when interviewing for people to join soft skills are a big thing?
Yeah, definitely. The skills you need for the consultancy part of the role, understanding the customers problems, are a bit different to the skills needed to be a good engineer - you need to be able to engage with the customers. Someone who can talk, who can do a presentation without worrying about it, that is really valuable.
Is there anything specific that you would look for or do you during the interview process to assess this?
This is giving the game away for any future interview processes, but when candidates come in we get them a drink, and they'll probably meet a senior member of the team who happens to be sitting near the coffee machine and asks simple questions, like:
Who are you? What do you do? What's your background? Where have you come from today?
So we drop into a friendly conversation, see if they can carry a conversation with someone senior. And we also have an interview stage where we ask them to do a presentation on some work that they've done in the past. Again, it's assessing how they communicate to people who aren’t experts in the area, and if they can get it across and make the audience feel like they know what they're talking about. Some people will get stressed about that, and that's probably a sign they might be really good on the machine learning side, but the soft skills aren’t where we need them to be for us.
If you're seeking top talent in the field of MLOps or looking for exciting career opportunities, don't hesitate to get in touch with our experienced AI & ML recruitment team. We connect businesses with skilled professionals and provide a platform for individuals to explore promising MLOps jobs. Whether you're looking to hire talent, browse job listings, or even feature in our blog series, we're here to facilitate your journey in the world of MLOps. Reach out to us today to learn more!